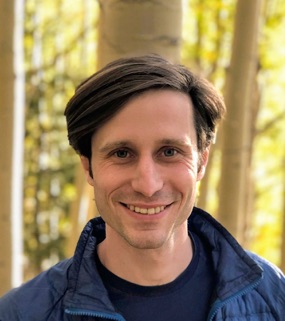
Everyday hearing – understanding an utterance, recognizing a voice, or picking out the melody of a song – is a feat of biological engineering. Machine hearing systems are just beginning to catch-up to their biological counterparts and still lag behind them in many respects. The broad goal of my research is to understand the neural computations that underlie human hearing, crystallize these insights in computational models, and use these models to improve machine systems and understand hearing impairments.
Below are some of the topics and questions that I’m working on:
Organization of human auditory cortex. The early stages of the auditory system have been extensively characterized in animals, but less is known about later cortical stages, whose responses likely underlie our perception and may be specific to humans. I’m trying to figure out how responses in these higher-order regions are organized, how they differ from those in earlier stages, how they develop and change, and what computational principles underlie their response. I’m also collaborating with animal researchers to figure out how human cortical responses differ from those in other species.
Temporal integration. Sound is a temporal signal, and temporal integration is thus an inevitable and essential part of audition. To derive meaning from natural sounds like speech, the brain must rapidly recognize, remember, and synthesize information across an enormous range of timescales spanning tens (e.g. phonemes), hundreds (e.g. words), and thousands of milliseconds (e.g. sentence). I’m developing a variety of experimental and computational methods to understand how the human auditory cortex accomplishes this remarkable feat.
Exploiting deep learning. Brain responses are often impossible to understand without some kind of model that links those responses to the behaviors they support. I’m using deep learning – which provides a general-purpose toolkit for training models on challenging real-world tasks – to make sense of the responses we observe in higher-order regions of human auditory cortex. A part of this work involves developing better methods for comparing brain responses with those from complex models like deep networks.
Source separation. Both neuroscientists and engineers tend to think of sensory stimuli as a collection of features (e.g. frequency, pitch). But perception is fundamentally organized around objects or in the case of audition ‘sources’, such as a person talking, an instrument playing, or stream babbling. I’m developing models that can learn features that are by construction easy to group into their underlying sources, providing a plausible model for how sound sources are represented in the brain.
Experimental and computational methods. Natural stimuli are complex and high-dimensional, which is what makes understanding their neural representation both essential and challenging. I have been working on experimental and computational methods to study the neural coding of natural sounds. Examples include: (1) statistical methods to reveal brain organization from responses to natural stimuli (2) a synthesis method to test if a computational model explains neural responses to natural stimuli (3) an experimental paradigm to infer the time window over which a neural response integrates information in natural stimuli.
Background
I was drawn to cognitive science by the intelligence of perception. I studied vision as an undergraduate at Yale (with Nick Turk-Browne, Gregory McCarthy, and Marvin Chun), and then became interested in the neural mechanisms of hearing as a graduate student at MIT, where I was trained in computational perception and cognitive neuroscience by Josh McDermott and Nancy Kanwisher. Since receiving my PhD, I have been been largely independent thanks to a research fellowship from the HHMI / Life Sciences Research Foundation and a recent K99/R00 award. I have collaborated with animal researchers (Bevil Conway, Shihab Shamma, and Yves Boubenec) to compare the functional organization of human auditory cortex with those of other species. And for the past year, I have been working with Nima Mesgarani at Columbia University to study how auditory cortex integrates temporal information in natural sounds, using intracranial recordings from human patients.
Press coverage
NPR on Comparing Human and Monkey Tone Responses
New York Times on Music Selective Responses
E-mail me (sn2776@columbia.edu) if you have any questions, or are interested in learning more about my research.