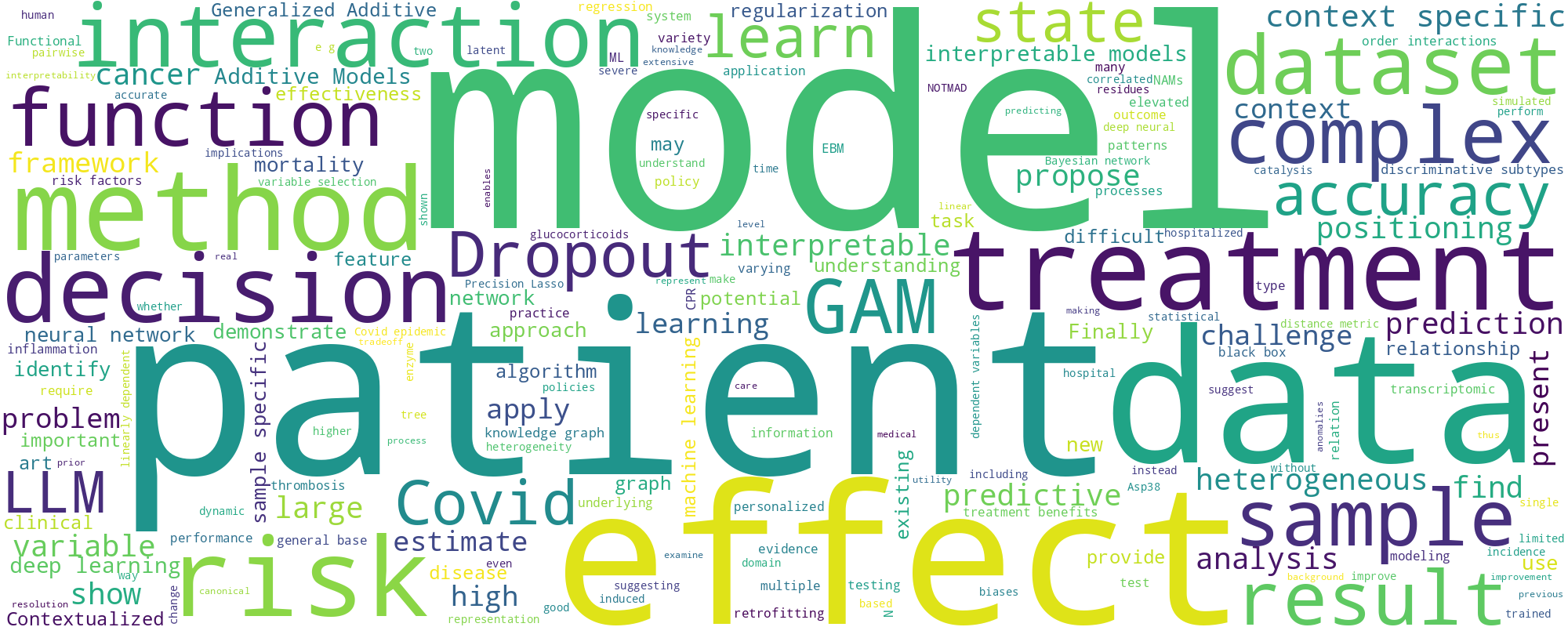
Research Interests and Selected Publications
-
Context-Adaptive Systems (Meta- and Contextualized Learning): How do we build AI agents that adapt to context?
Selected Publications-
Contextualized Machine LearningBen Lengerich, Caleb Ellington, Andrea Rubbi, Manolis Kellis, Eric XingAbstract Pre-print
We examine Contextualized Machine Learning (ML), a paradigm for learning heterogeneous and context-dependent effects. Contextualized ML estimates heterogeneous functions by applying deep learning to the meta-relationship between contextual information and context-specific parametric models. This is a form of varying-coefficient modeling that unifies existing frameworks including cluster analysis and cohort modeling by introducing two reusable concepts: a context encoder which translates sample context into model parameters, and sample-specific model which operates on sample predictors. We review the process of developing contextualized models, nonparametric inference from contextualized models, and identifiability conditions of contextualized models. Finally, we present the open-source PyTorch package ContextualizedML.
arXiv 2023 Code: Contextualized.ML Python package
Talk: ContextualizedML for Disease Subtyping (presented at BIRS). Video/slides available.
-
NOTMAD: Estimating Bayesian Networks with Sample-Specific Structures and ParametersBenjamin Lengerich, Caleb Ellington, Bryon Aragam, Eric P. Xing, Manolis KellisAbstract Pre-print
Context-specific Bayesian networks (i.e. directed acyclic graphs, DAGs) identify context-dependent relationships between variables, but the non-convexity induced by the acyclicity requirement makes it difficult to share information between context-specific estimators (e.g. with graph generator functions). For this reason, existing methods for inferring context-specific Bayesian networks have favored breaking datasets into subsamples, limiting statistical power and resolution, and preventing the use of multidimensional and latent contexts. To overcome this challenge, we propose NOTEARS-optimized Mixtures of Archetypal DAGs (NOTMAD). NOTMAD models context-specific Bayesian networks as the output of a function which learns to mix archetypal networks according to sample context. The archetypal networks are estimated jointly with the context-specific networks and do not require any prior knowledge. We encode the acyclicity constraint as a smooth regularization loss which is back-propagated to the mixing function; in this way, NOTMAD shares information between context-specific acyclic graphs, enabling the estimation of Bayesian network structures and parameters at even single-sample resolution. We demonstrate the utility of NOTMAD and sample-specific network inference through analysis and experiments, including patient-specific gene expression networks which correspond to morphological variation in cancer.
arXiv 2022
-
-
Prior Knowledge as Context: Connecting Statistical Inference to Foundation Models
Selected Publications-
LLMs Understand Glass-Box Models, Discover Surprises, and Suggest RepairsBenjamin J. Lengerich, Sebastian Bordt, Harsha Nori, Mark E. Nunnally, Yin Aphinyanaphongs, Manolis Kellis, Rich CaruanaAbstract Pre-print
We show that large language models (LLMs) are remarkably good at working with interpretable models that decompose complex outcomes into univariate graph-represented components. By adopting a hierarchical approach to reasoning, LLMs can provide comprehensive model-level summaries without ever requiring the entire model to fit in context. This approach enables LLMs to apply their extensive background knowledge to automate common tasks in data science such as detecting anomalies that contradict prior knowledge, describing potential reasons for the anomalies, and suggesting repairs that would remove the anomalies. We use multiple examples in healthcare to demonstrate the utility of these new capabilities of LLMs, with particular emphasis on Generalized Additive Models (GAMs). Finally, we present the package TalkToEBM as an open-source LLM-GAM interface.
arXiv 2023 Code: TalkToEBM Python package
-
Retrofitting Distributional Embeddings to Knowledge Graphs with Functional RelationsBenjamin J. Lengerich, Andrew Maas, Christopher PottsAbstract Paper Pre-print
Knowledge graphs are a versatile framework to encode richly structured data relationships, but it can be challenging to combine these graphs with unstructured data. Methods for retrofitting pre-trained entity representations to the structure of a knowledge graph typically assume that entities are embedded in a connected space and that relations imply similarity. However, useful knowledge graphs often contain diverse entities and relations (with potentially disjoint underlying corpora) which do not accord with these assumptions. To overcome these limitations, we present Functional Retrofitting, a framework that generalizes current retrofitting methods by explicitly modeling pairwise relations. Our framework can directly incorporate a variety of pairwise penalty functions previously developed for knowledge graph completion. Further, it allows users to encode, learn, and extract information about relation semantics. We present both linear and neural instantiations of the framework. Functional Retrofitting significantly outperforms existing retrofitting methods on complex knowledge graphs and loses no accuracy on simpler graphs (in which relations do imply similarity). Finally, we demonstrate the utility of the framework by predicting new drug--disease treatment pairs in a large, complex health knowledge graph.
COLING 2018
-
-
Interpretable Representations of Complex and Nonlinear Systems: How can we build models that summarize complicated patterns in interpretable ways?
Selected Publications-
Neural Additive Models: Interpretable Machine Learning with Neural NetsRishabh Agarwal, Levi Melnick, Nicholas Frosst, Xuezhou Zhang, Ben Lengerich, Rich Caruana, Geoffrey E HintonAbstract Paper Pre-print
Deep neural networks (DNNs) are powerful black-box predictors that have achieved impressive performance on a wide variety of tasks. However, their accuracy comes at the cost of intelligibility: it is usually unclear how they make their decisions. This hinders their applicability to high stakes decision-making domains such as healthcare. We propose Neural Additive Models (NAMs) which combine some of the expressivity of DNNs with the inherent intelligibility of generalized additive models. NAMs learn a linear combination of neural networks that each attend to a single input feature. These networks are trained jointly and can learn arbitrarily complex relationships between their input feature and the output. Our experiments on regression and classification datasets show that NAMs are more accurate than widely used intelligible models such as logistic regression and shallow decision trees. They perform similarly to existing state-of-the-art generalized additive models in accuracy, but are more flexible because they are based on neural nets instead of boosted trees. To demonstrate this, we show how NAMs can be used for multitask learning on synthetic data and on the COMPAS recidivism data due to their composability, and demonstrate that the differentiability of NAMs allows them to train more complex interpretable models for COVID-19.
NeurIPS 2021 -
Purifying Interaction Effects with the Functional ANOVA: An Efficient Algorithm for Recovering Identifiable Additive ModelsBenjamin Lengerich, Sarah Tan, Chun-Hao Chang, Giles Hooker, Rich CaruanaAbstract Paper Pre-print
Models which estimate main effects of individual variables alongside interaction effects have an identifiability challenge: effects can be freely moved between main effects and interaction effects without changing the model prediction. This is a critical problem for interpretability because it permits “contradictory" models to represent the same function. To solve this problem, we propose pure interaction effects: variance in the outcome which cannot be represented by any subset of features. This definition has an equivalence with the Functional ANOVA decomposition. To compute this decomposition, we present a fast, exact algorithm that transforms any piecewise-constant function (such as a tree-based model) into a purified, canonical representation. We apply this algorithm to Generalized Additive Models with interactions trained on several datasets and show large disparity, including contradictions, between the apparent and the purified effects. These results underscore the need to specify data distributions and ensure identifiability before interpreting model parameters.
AISTATS 2020 Code: Interpret.ML Python package.
-
-
Clinical Tools for Personalized Medicine: How can we analyze real-world evidence to improve care for every patient?
Selected Publications-
Death by Round Numbers and Sharp Thresholds: Glass-Box Machine Learning Uncovers Biases in Medical PracticeBenjamin Lengerich, Rich Caruana, Mark E. Nunnally, Manolis KellisAbstract Pre-print
Real-world evidence is confounded by treatments, so data-driven systems can learn to recapitulate biases that influenced treatment decisions. This confounding presents a challenge: uninterpretable black-box systems can put patients at risk by confusing treatment benefits with intrinsic risk, but also an opportunity: interpretable “glass-box” models can improve medical practice by highlighting unexpected patterns which suggest biases in medical practice. We propose a glass-box model that enables clinical experts to find unexpected changes in patient mortality risk. By applying this model to four datasets, we identify two characteristic types of biases: (1) discontinuities where sharp treatment thresholds produce step-function changes in risk near clinically-important round-number cutoffs, and (2) counter-causal paradoxes where aggressive treatment produces non-monotone risk curves that contradict underlying causal risk by lowering the risk of treated patients below that of healthier, but untreated, patients. While these effects are learned by all accurate models, they are only revealed by interpretable models. We show that because these effects are the result of clinical practice rather than statistical aberration, they are pervasive even in large, canonical datasets. Finally, we apply this method to uncover opportunities for improvements in clinical practice, including 8000 excess deaths per year in the US, where paradoxically, patients with moderately-elevated serum creatinine have higher mortality risk than patients with severely-elevated serum creatinine.
medrxiv 2022 -
Automated interpretable discovery of heterogeneous treatment effectiveness: A COVID-19 case studyBenjamin J Lengerich, Mark E Nunnally, Yin Aphinyanaphongs, Caleb Ellington, Rich CaruanaAbstract Paper Pre-print
Testing multiple treatments for heterogeneous (varying) effectiveness with respect to many underlying risk factors requires many pairwise tests; we would like to instead automatically discover and visualize patient archetypes and predictors of treatment effectiveness using multitask machine learning. In this paper, we present a method to estimate these heterogeneous treatment effects with an interpretable hierarchical framework that uses additive models to visualize expected treatment benefits as a function of patient factors (identifying personalized treatment benefits) and concurrent treatments (identifying combinatorial treatment benefits). This method achieves state-of-the-art predictive power for COVID-19 in-hospital mortality and interpretable identification of heterogeneous treatment benefits. We first validate this method on the large public MIMIC-IV dataset of ICU patients to test recovery of heterogeneous treatment effects. Next we apply this method to a proprietary dataset of over 3000 patients hospitalized for COVID-19, and find evidence of heterogeneous treatment effectiveness predicted largely by indicators of inflammation and thrombosis risk: patients with few indicators of thrombosis risk benefit most from treatments against inflammation, while patients with few indicators of inflammation risk benefit most from treatments against thrombosis. This approach provides an automated methodology to discover heterogeneous and individualized effectiveness of treatments.
JBI 2022 -
Interpretable Predictive Models to Understand Risk Factors for Maternal and Fetal OutcomesTomas M. Bosschieter, Zifei Xu, Hui Lan, Benjamin J. Lengerich, Harsha Nori, Ian Painter, Vivienne Souter, Rich CaruanaAbstract Paper
Although most pregnancies result in a good outcome, complications are not uncommon and can be associated with serious implications for mothers and babies. Predictive modeling has the potential to improve outcomes through a better understanding of risk factors, heightened surveillance for high-risk patients, and more timely and appropriate interventions, thereby helping obstetricians deliver better care. We identify and study the most important risk factors for four types of pregnancy complications: (i) severe maternal morbidity, (ii) shoulder dystocia, (iii) preterm preeclampsia, and (iv) antepartum stillbirth. We use an Explainable Boosting Machine (EBM), a high-accuracy glass-box learning method, for the prediction and identification of important risk factors. We undertake external validation and perform an extensive robustness analysis of the EBM models. EBMs match the accuracy of other black-box ML methods, such as deep neural networks and random forests, and outperform logistic regression, while being more interpretable. EBMs prove to be robust. The interpretability of the EBM models reveal surprising insights into the features contributing to risk (e.g., maternal height is the second most important feature for shoulder dystocia) and may have potential for clinical application in the prediction and prevention of serious complications in pregnancy.
JHIR 2023
-
Active Projects
-
Contextualized Learning
Details- Reformulating all sorts of models in computational biology and precision medicine for contextualized inference.
- Statistical identifiability and robustness of context-specific models.
- Efficient inference of contextualized models in partially-observed and active environments.
-
Contextualized Effects of Complex Disease
Details- Cross-disease analysis of Alzheimer's disease and Down syndrome.
- Ancestry-specific effects, biomarkers, and therapeutic targets in metabolic disorders.
- Personalized subtyping of cancer, including network-based subtyping using latent gene regulatory networks.
-
Connecting Statistical Inference to Foundation Models
Details- How can we use prior knowledge to improve statistical inference?
- How can we use statistical inference to improve foundation models?
- How can we integrate all kinds of data, including knowledge graphs, foundation models, and observational data?
-
Automated analysis of real-world evidence
Details- Can we automatically identify hidden confounders in RWE by looking for characteristic patterns?
- Are LLMs better than domain experts at interpreting statistical models in context?
- How can automated discovery of hidden confounders in RWE help us improve medical practice?
-
Clinical Tools
Details- Can we predict the risk of adverse outcomes in pregnancy, especially labor and delivery, at times appropriate for early intervention?
- Automatically organizing information to assist clinician workloads.